Practice Free DP-100 Exam Online Questions
HOTSPOT
You are the owner of an Azure Machine Learning workspace.
You must prevent the creation or deletion of compute resources by using a custom role. You must allow all other operations inside the workspace.
You need to configure the custom role.
How should you complete the configuration? To answer, select the appropriate options in the answer area. NOTE: Each correct selection is worth one point.
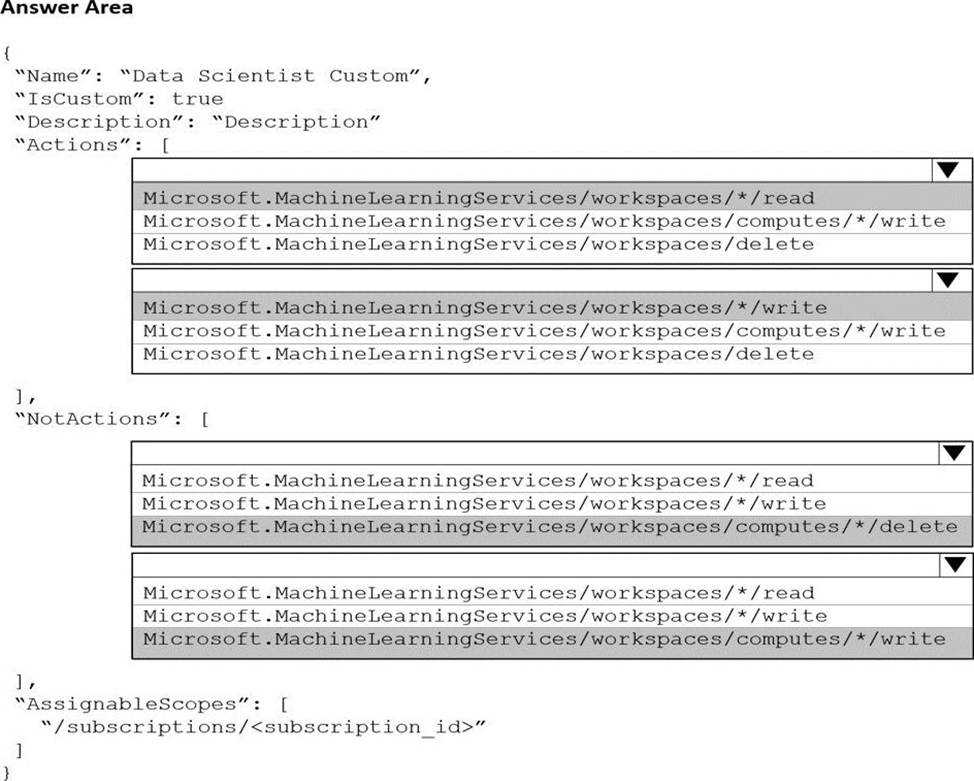
Explanation:
Box 1: Microsoft.MachineLearningServices/workspaces/*/read
Reader role: Read-only actions in the workspace. Readers can list and view assets, including datastore credentials, in a workspace. Readers can’t create or update these assets.
Box 2: Microsoft.MachineLearningServices/workspaces/*/write
If the roles include Actions that have a wildcard (*), the effective permissions are computed by subtracting the NotActions from the allowed Actions.
Box 3: Box 2: Microsoft.MachineLearningServices/workspaces/computes/*/delete
Box 4: Microsoft.MachineLearningServices/workspaces/computes/*/write
Reference: https://docs.microsoft.com/en-us/azure/role-based-access-control/overview#how-azure-rbac-determines-if-a-user-has-access-to-a-resource
HOTSPOT
You create a Python script named train.py and save it in a folder named scripts. The script uses the scikit-learn framework to train a machine learning model.
You must run the script as an Azure Machine Learning experiment on your local workstation.
You need to write Python code to initiate an experiment that runs the train.py script.
How should you complete the code segment? To answer, select the appropriate options in the answer area. NOTE: Each correct selection is worth one point.
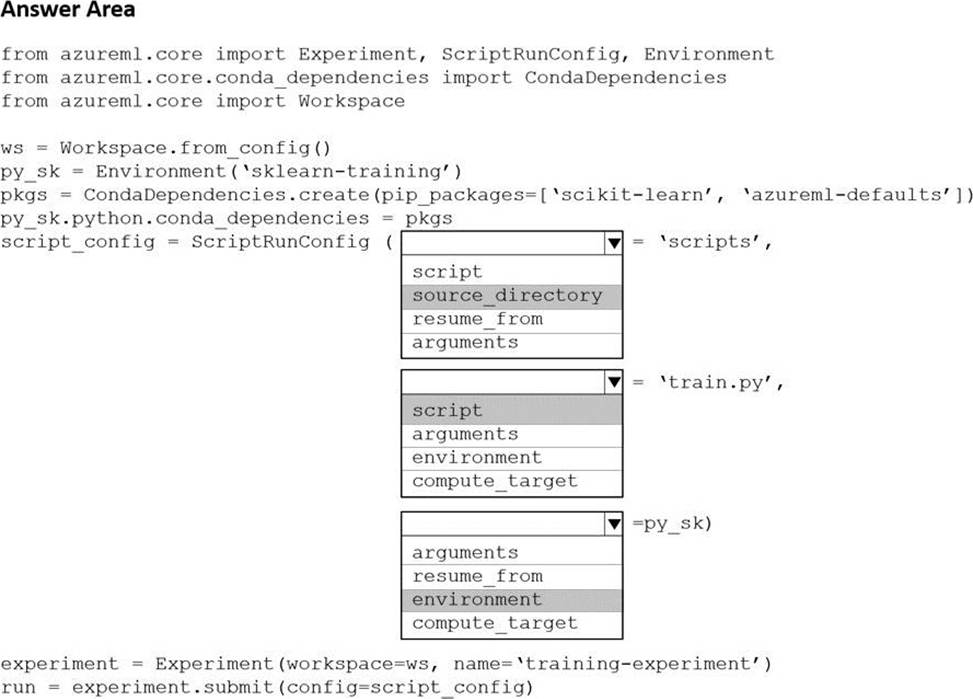
Explanation:
Box 1: source_directory
source_directory: A local directory containing code files needed for a run.
Box 2: script
Script: The file path relative to the source_directory of the script to be run.
Box 3: environment
Reference: https://docs.microsoft.com/en-us/python/api/azureml-core/azureml.core.scriptrunconfig
You are implementing a machine learning model to predict stock prices.
The model uses a PostgreSQL database and requires GPU processing.
You need to create a virtual machine that is pre-configured with the required tools.
What should you do?
- A . Create a Data Science Virtual Machine (DSVM) Windows edition.
- B . Create a Geo Al Data Science Virtual Machine (Geo-DSVM) Windows edition.
- C . Create a Deep Learning Virtual Machine (DLVM) Linux edition.
- D . Create a Deep Learning Virtual Machine (DLVM) Windows edition.
- E . Create a Data Science Virtual Machine (DSVM) Linux edition.
E
Explanation:
Incorrect Answers:
A, C: PostgreSQL (CentOS) is only available in the Linux Edition.
B: The Azure Geo AI Data Science VM (Geo-DSVM) delivers geospatial analytics capabilities from Microsoft’s
Data Science VM. Specifically, this VM extends the AI and data science toolkits in the Data Science VM by adding ESRI’s market-leading ArcGIS Pro Geographic Information System.
D: DLVM is a template on top of DSVM image. In terms of the packages, GPU drivers etc are all there in the DSVM image. Mostly it is for convenience during creation where we only allow DLVM to be created on GPU VM instances on Azure.
Reference: https://docs.microsoft.com/en-us/azure/machine-learning/data-science-virtual-machine/overview
You plan to use a Deep Learning Virtual Machine (DLVM) to train deep learning models using Compute Unified Device Architecture (CUDA) computations.
You need to configure the DLVM to support CUDA.
What should you implement?
- A . Intel Software Guard Extensions (Intel SGX) technology
- B . Solid State Drives (SSD)
- C . Graphic Processing Unit (GPU)
- D . Computer Processing Unit (CPU) speed increase by using overcloking
- E . High Random Access Memory (RAM) configuration
C
Explanation:
A Deep Learning Virtual Machine is a pre-configured environment for deep learning using GPU instances.
Reference: https://azuremarketplace.microsoft.com/en-au/marketplace/apps/microsoft-ads.dsvm-deep-learning
Note: This question is part of a series of questions that present the same scenario. Each question in the series contains a unique solution that might meet the stated goals. Some question sets might have more than one correct solution, while others might not have a correct solution.
After you answer a question in this section, you will NOT be able to return to it. As a result, these questions will not appear in the review screen.
You use Azure Machine Learning designer to load the following datasets into an experiment:
You need to create a dataset that has the same columns and header row as the input datasets and contains all rows from both input datasets.
Solution: Use the Join Data module.
Does the solution meet the goal?
- A . Yes
- B . No
Note: This question is part of a series of questions that present the same scenario. Each question in the series contains a unique solution that might meet the stated goals. Some question sets might have more than one correct solution, while others might not have a correct solution.
After you answer a question in this section, you will NOT be able to return to it. As a result, these questions will not appear in the review screen.
You use Azure Machine Learning designer to load the following datasets into an experiment:
You need to create a dataset that has the same columns and header row as the input datasets and contains all rows from both input datasets.
Solution: Use the Add Rows module.
Does the solution meet the goal?
- A . Yes
- B . No
You create an MLflow model
You must deploy the model to Azure Machine Learning for batch inference.
You need to create the batch deployment.
Which two components should you use? Each correct answer presents a complete solution. NOTE: Each correct selection is worth one point
- A . Compute target
- B . Kubernetes online endpoint
- C . Model files
- D . Online endpoint
- E . Environment
Note: This question is part of a series of questions that present the same scenario. Each question in the series contains a unique solution that might meet the stated goals. Some question sets might have more than one correct solution, while others might not have a correct solution.
After you answer a question in this section, you will NOT be able to return to it. As a result, these questions will not appear in the review screen.
You plan to use a Python script to run an Azure Machine Learning experiment.
The script creates a reference to the experiment run context, loads data from a file, identifies the set of unique values for the label column, and completes the experiment run:
from azureml.core import Run
import pandas as pd
run = Run.get_context()
data = pd.read_csv(‘data.csv’)
label_vals = data[‘label’].unique()
# Add code to record metrics here
run.complete()
The experiment must record the unique labels in the data as metrics for the run that can be reviewed later.
You must add code to the script to record the unique label values as run metrics at the point indicated by the comment.
Solution: Replace the comment with the following code:
run.log_list(‘Label Values’, label_vals)
Does the solution meet the goal?
- A . Yes
- B . No
A
Explanation:
run.log_list log a list of values to the run with the given name using log_list.
Example: run.log_list("accuracies", [0.6, 0.7, 0.87])
Note:
Data= pd.read_csv(‘data.csv’)
Data is read into a pandas.DataFrame, which is a two-dimensional, size-mutable, potentially heterogeneous tabular data.
label_vals =data[‘label’].unique
label_vals contains a list of unique label values.
Reference:
https://www.element61.be/en/resource/azure-machine-learning-services-complete-toolbox-ai
https://docs.microsoft.com/en-us/python/api/azureml-core/azureml.core.run(class)
https://pandas.pydata.org/docs/reference/api/pandas.DataFrame.html
You manage an Azure Machine Learning workspace. You design a training job that is configured with a serverless compute. The serverless compute must have a specific instance type and count
You need to configure the serverless compute by using Azure Machine Learning Python SDK v2.
What should you do?
- A . Specify the compute name by using the compute parameter of the command job
- B . Configure the tier parameter to Dedicated VM.
- C . Initialize and specify the ResourceConfiguration class
- D . Initialize AmICompute class with size and type specification.
HOTSPOT
You manage an Azure Machine Learning workspace by using the Python SDK v2.
You must create a compute cluster in the workspace. The compute cluster must run workloads and properly handle interruptions. You start by calculating the maximum amount of compute resources required by the workloads and size the cluster to match the calculations.
The cluster definition includes the following properties and values:
• name="mlcluster1’’
• size="STANDARD.DS3.v2"
• min_instances=1
• maxjnstances=4
• tier="dedicated"
The cost of the compute resources must be minimized when a workload is active Of idle. Cluster property changes must not affect the maximum amount of compute resources available to the workloads run on the cluster.
You need to modify the cluster properties to minimize the cost of compute resources.
Which properties should you modify? To answer, select the appropriate options in the answer area. NOTE: Each correct selection is worth one point.
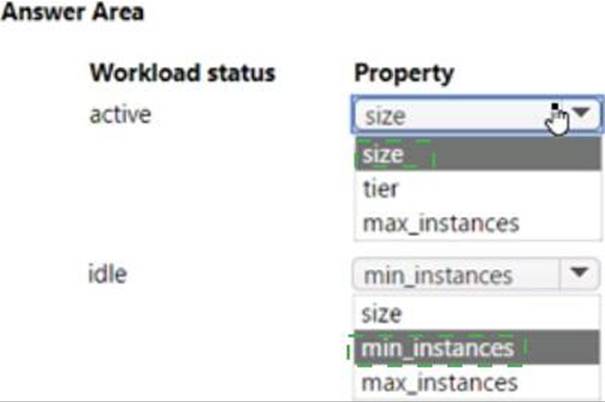