Practice Free DP-100 Exam Online Questions
HOTSPOT
You create an Azure Machine Learning compute target named ComputeOne by using the STANDARD_D1 virtual machine image.
You define a Python variable named was that references the Azure Machine Learning workspace.
You run the following Python code:
For each of the following statements, select Yes if the statement is true. Otherwise, select No. NOTE: Each correct selection is worth one point.
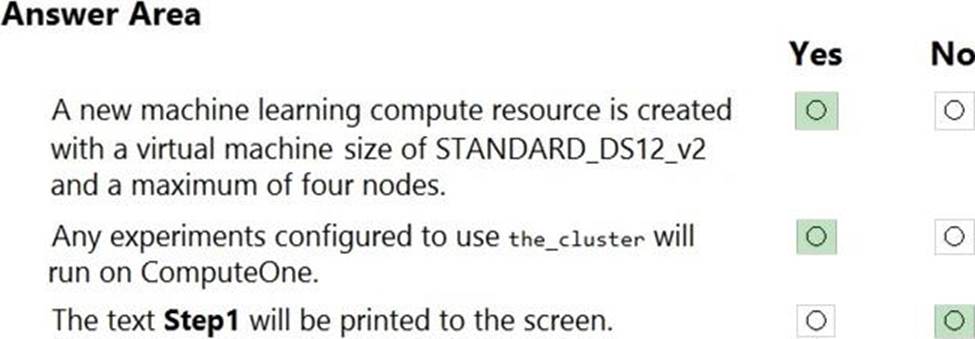
Explanation:
Box 1: Yes
ComputeTargetException class: An exception related to failures when creating, interacting with, or configuring a compute target. This exception is commonly raised for failures attaching a compute target, missing headers, and unsupported configuration values.
Create (workspace, name, provisioning_configuration)
Provision a Compute object by specifying a compute type and related configuration.
This method creates a new compute target rather than attaching an existing one.
Box 2: Yes
Box 3: No
The line before print(‘Step1’) will fail.
Reference: https://docs.microsoft.com/en-us/python/api/azureml-core/azureml.core.compute.computetarget
You create a deep learning model for image recognition on Azure Machine Learning service using GPU-based training.
You must deploy the model to a context that allows for real-time GPU-based inferencing.
You need to configure compute resources for model inferencing.
Which compute type should you use?
- A . Azure Container Instance
- B . Azure Kubernetes Service
- C . Field Programmable Gate Array
- D . Machine Learning Compute
B
Explanation:
You can use Azure Machine Learning to deploy a GPU-enabled model as a web service. Deploying a model on Azure Kubernetes Service (AKS) is one option. The AKS cluster provides a GPU resource that is used by the model for inference.
Inference, or model scoring, is the phase where the deployed model is used to make predictions.
Using GPUs instead of CPUs offers performance advantages on highly parallelizable computation.
Reference: https://docs.microsoft.com/en-us/azure/machine-learning/how-to-deploy-inferencing-gpus
HOTSPOT
You are a lead data scientist for a project that tracks the health and migration of birds. You create a multi-image classification deep learning model that uses a set of labeled bird photos collected by experts. You plan to use the model to develop a cross-platform mobile app that predicts the species of bird captured by app users.
You must test and deploy the trained model as a web service.
The deployed model must meet the following requirements:
✑ An authenticated connection must not be required for testing.
✑ The deployed model must perform with low latency during inferencing.
✑ The REST endpoints must be scalable and should have a capacity to handle large number of requests when multiple end users are using the mobile application.
You need to verify that the web service returns predictions in the expected JSON format when a valid REST request is submitted.
Which compute resources should you use? To answer, select the appropriate options in the answer area. NOTE: Each correct selection is worth one point.
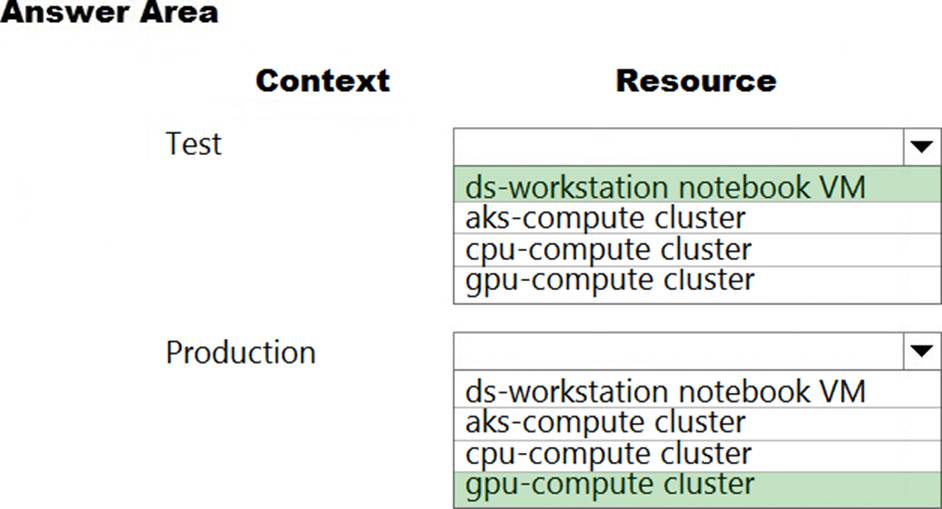
Explanation:
Box 1: ds-workstation notebook VM
An authenticated connection must not be required for testing.
On a Microsoft Azure virtual machine (VM), including a Data Science Virtual Machine (DSVM), you create local user accounts while provisioning the VM. Users then authenticate to the VM by using these credentials.
Box 2: gpu-compute cluster
Image classification is well suited for GPU compute clusters
Reference:
https://docs.microsoft.com/en-us/azure/machine-learning/data-science-virtual-machine/dsvm-common-identity
https://docs.microsoft.com/en-us/azure/architecture/reference-architectures/ai/training-deep-learning
You create a binary classification model by using Azure Machine Learning Studio.
You must tune hyperparameters by performing a parameter sweep of the model.
The parameter sweep must meet the following requirements:
iterate all possible combinations of hyperparameters minimize computing resources required to perform the sweep You need to perform a parameter sweep of the model.
Which parameter sweep mode should you use?
- A . Random sweep
- B . Sweep clustering
- C . Entire grid
- D . Random grid
- E . Random seed
D
Explanation:
Maximum number of runs on random grid: This option also controls the number of iterations over a random sampling of parameter values, but the values are not generated randomly from the specified range; instead, a matrix is created of all possible combinations of parameter values and a random sampling is taken over the matrix. This method is more efficient and less prone to regional oversampling or undersampling.
If you are training a model that supports an integrated parameter sweep, you can also set a range of seed values to use and iterate over the random seeds as well. This is optional, but can be useful for avoiding bias introduced by seed selection.
Incorrect Answers:
B: If you are building a clustering model, use Sweep Clustering to automatically determine the optimum number of clusters and other parameters.
C: Entire grid: When you select this option, the module loops over a grid predefined by the system, to try different combinations and identify the best learner. This option is useful for cases where you don’t know what the best parameter settings might be and want to try all possible combination of values.
E: If you choose a random sweep, you can specify how many times the model should be trained, using a random combination of parameter values.
Reference: https://docs.microsoft.com/en-us/azure/machine-learning/studio-module-reference/tune-model-hyperparameters
You are solving a classification task.
The dataset is imbalanced.
You need to select an Azure Machine Learning Studio module to improve the classification accuracy.
Which module should you use?
- A . Fisher Linear Discriminant Analysis.
- B . Filter Based Feature Selection
- C . Synthetic Minority Oversampling Technique (SMOTE)
- D . Permutation Feature Importance
C
Explanation:
Use the SMOTE module in Azure Machine Learning Studio (classic) to increase the number of underepresented cases in a dataset used for machine learning. SMOTE is a better way of increasing the number of rare cases than simply duplicating existing cases.
You connect the SMOTE module to a dataset that is imbalanced. There are many reasons why a dataset might be imbalanced: the category you are targeting might be very rare in the population, or the data might simply be difficult to collect. Typically, you use SMOTE when the class you want to analyze is under-represented.
Reference: https://docs.microsoft.com/en-us/azure/machine-learning/studio-module-reference/smote
You are solving a classification task.
The dataset is imbalanced.
You need to select an Azure Machine Learning Studio module to improve the classification accuracy.
Which module should you use?
- A . Fisher Linear Discriminant Analysis.
- B . Filter Based Feature Selection
- C . Synthetic Minority Oversampling Technique (SMOTE)
- D . Permutation Feature Importance
C
Explanation:
Use the SMOTE module in Azure Machine Learning Studio (classic) to increase the number of underepresented cases in a dataset used for machine learning. SMOTE is a better way of increasing the number of rare cases than simply duplicating existing cases.
You connect the SMOTE module to a dataset that is imbalanced. There are many reasons why a dataset might be imbalanced: the category you are targeting might be very rare in the population, or the data might simply be difficult to collect. Typically, you use SMOTE when the class you want to analyze is under-represented.
Reference: https://docs.microsoft.com/en-us/azure/machine-learning/studio-module-reference/smote
You use Azure Machine Learning Designer lo load the following datasets into an experiment:
Dataset1:
Dataset2:
You need to create a dataset that has the same columns and header row as the input datasets and contains all rows from both input datasets.
Solution: Use the Add Rows component.
Does the solution meet the goal?
- A . Yes
- B . No
You use Azure Machine Learning Designer lo load the following datasets into an experiment:
Dataset1:
Dataset2:
You need to create a dataset that has the same columns and header row as the input datasets and contains all rows from both input datasets.
Solution: Use the Add Rows component.
Does the solution meet the goal?
- A . Yes
- B . No
Note: This question is part of a series of questions that present the same scenario. Each question in the series contains a unique solution that might meet the stated goals. Some question sets might have more than one correct solution, while others might not have a correct solution.
After you answer a question in this section, you will NOT be able to return to it. As a result, these questions will not appear in the review screen.
You are using Azure Machine Learning Studio to perform feature engineering on a dataset.
You need to normalize values to produce a feature column grouped into bins.
Solution: Apply an Entropy Minimum Description Length (MDL) binning mode.
Does the solution meet the goal?
- A . Yes
- B . No
A
Explanation:
Entropy MDL binning mode: This method requires that you select the column you want to predict and the column or columns that you want to group into bins. It then makes a pass over the data and attempts to determine the number of bins that minimizes the entropy. In other words, it chooses a number of bins that allows the data column to best predict the target column. It then returns the bin number associated with each row of your data in a column named <colname>quantized.
Reference: https://docs.microsoft.com/en-us/azure/machine-learning/studio-module-reference/group-data-into-bins
Note: This question is part of a series of questions that present the same scenario. Each question in the series contains a unique solution that might meet the stated goals. Some question sets might have more than one correct solution, while others might not have a correct solution.
After you answer a question in this section, you will NOT be able to return to it. As a result, these questions will not appear in the review screen.
You are using Azure Machine Learning Studio to perform feature engineering on a dataset.
You need to normalize values to produce a feature column grouped into bins.
Solution: Apply an Entropy Minimum Description Length (MDL) binning mode.
Does the solution meet the goal?
- A . Yes
- B . No
A
Explanation:
Entropy MDL binning mode: This method requires that you select the column you want to predict and the column or columns that you want to group into bins. It then makes a pass over the data and attempts to determine the number of bins that minimizes the entropy. In other words, it chooses a number of bins that allows the data column to best predict the target column. It then returns the bin number associated with each row of your data in a column named <colname>quantized.
Reference: https://docs.microsoft.com/en-us/azure/machine-learning/studio-module-reference/group-data-into-bins