Practice Free DP-100 Exam Online Questions
You write a Python script that processes data in a comma-separated values (CSV) file.
You plan to run this script as an Azure Machine Learning experiment.
The script loads the data and determines the number of rows it contains using the following code:
You need to record the row count as a metric named row_count that can be returned using the get_metrics method of the Run object after the experiment run completes.
Which code should you use?
- A . run.upload_file(‘row_count’, ‘./data.csv’)
- B . run.log(‘row_count’, rows)
- C . run.tag(‘row_count’, rows)
- D . run.log_table(‘row_count’, rows)
- E . run.log_row(‘row_count’, rows)
B
Explanation:
Log a numerical or string value to the run with the given name using log(name, value, description=”). Logging a metric to a run causes that metric to be stored in the run record in the experiment. You can log the same metric multiple times within a run, the result being considered a vector of that metric.
Example: run.log("accuracy", 0.95)
Incorrect Answers:
E: Using log_row(name, description=None, **kwargs) creates a metric with multiple columns as described in kwargs. Each named parameter generates a column with the value specified. log_row can be called once to log an arbitrary tuple, or multiple times in a loop to generate a complete table.
Example: run.log_row("Y over X", x=1, y=0.4)
Reference: https://docs.microsoft.com/en-us/python/api/azureml-core/azureml.core.run
You manage an Azure Machine Learning workspace. The development environment for managing the workspace is configured to use Python SDK v2 in Azure Machine Learning Notebooks
A Synapse Spark Compute is currently attached and uses system-assigned identity
You need to use Python code to update the Synapse Spark Compute to use a user-assigned identity.
Solution: Create an instance of the MICIient class.
Does the solution meet the goal?
- A . Yes
- B . No
Topic 2, Case Study 2
Case study
Overview
You are a data scientist for Fabrikam Residences, a company specializing in quality private and commercial property in the United States. Fabrikam Residences is considering expanding into Europe and has asked you to investigate prices for private residences in major European cities. You use Azure Machine Learning Studio to measure the median value of properties. You produce a regression model to predict property prices by using the Linear Regression and Bayesian Linear Regression modules.
Datasets
There are two datasets in CSV format that contain property details for two cities, London and Paris, with the following columns:
The two datasets have been added to Azure Machine Learning Studio as separate datasets and included as the starting point of the experiment.
Dataset issues
The AccessibilityToHighway column in both datasets contains missing values. The missing data must be replaced with new data so that it is modeled conditionally using the other variables in the data before filling in the missing values.
Columns in each dataset contain missing and null values. The dataset also contains many outliers. The Age column has a high proportion of outliers. You need to remove the rows that have outliers in the Age column. The MedianValue and AvgRoomsinHouse columns both hold data in numeric format. You need to select a feature selection algorithm to analyze the relationship between the two columns in more detail.
Model fit
The model shows signs of overfitting. You need to produce a more refined regression model that reduces the overfitting.
Experiment requirements
You must set up the experiment to cross-validate the Linear Regression and Bayesian Linear Regression modules to evaluate performance.
In each case, the predictor of the dataset is the column named MedianValue. An initial investigation showed that the datasets are identical in structure apart from the MedianValue column. The smaller Paris dataset contains the MedianValue in text format, whereas the larger London dataset contains the MedianValue in numerical format. You must ensure that the datatype of the MedianValue column of the Paris dataset matches the structure of the London dataset.
You must prioritize the columns of data for predicting the outcome. You must use non-parameters statistics to measure the relationships.
You must use a feature selection algorithm to analyze the relationship between the MedianValue and AvgRoomsinHouse columns.
Model training
Given a trained model and a test dataset, you need to compute the permutation feature importance scores of feature variables. You need to set up the Permutation Feature Importance module to select the correct metric to investigate the model’s accuracy and replicate the findings.
You want to configure hyperparameters in the model learning process to speed the learning phase by using hyperparameters. In addition, this configuration should cancel the lowest performing runs at each evaluation interval, thereby directing effort and resources towards models that are more likely to be successful.
You are concerned that the model might not efficiently use compute resources in hyperparameter tuning. You also are concerned that the model might prevent an increase in the overall tuning time. Therefore, you need to implement an early stopping criterion on models that provides savings without terminating promising jobs.
Testing
You must produce multiple partitions of a dataset based on sampling using the Partition and Sample module in Azure Machine Learning Studio. You must create three equal partitions for cross-validation. You must also configure the cross-validation process so that the rows in the test and training datasets are divided evenly by properties that are near each city’s main river. The data that identifies that a property is near a river is held in the column named NextToRiver. You want to complete this task before the data goes through the sampling process.
When you train a Linear Regression module using a property dataset that shows data for property prices for a large city, you need to determine the best features to use in a model. You can choose standard metrics provided to measure performance before and after the feature importance process completes. You must ensure that the distribution of the features across multiple training models is consistent.
Data visualization
You need to provide the test results to the Fabrikam Residences team. You create data visualizations to aid in presenting the results.
You must produce a Receiver Operating Characteristic (ROC) curve to conduct a
diagnostic test evaluation of the model. You need to select appropriate methods for producing the ROC curve in Azure Machine Learning Studio to compare the Two-Class Decision Forest and the Two-Class Decision Jungle modules with one another.
DRAG DROP
You need to implement early stopping criteria as suited in the model training requirements.
Which three code segments should you use to develop the solution? To answer, move the appropriate code segments from the list of code segments to the answer area and arrange them in the correct order. NOTE: More than one order of answer choices is correct. You will receive credit for any of the correct orders you select.
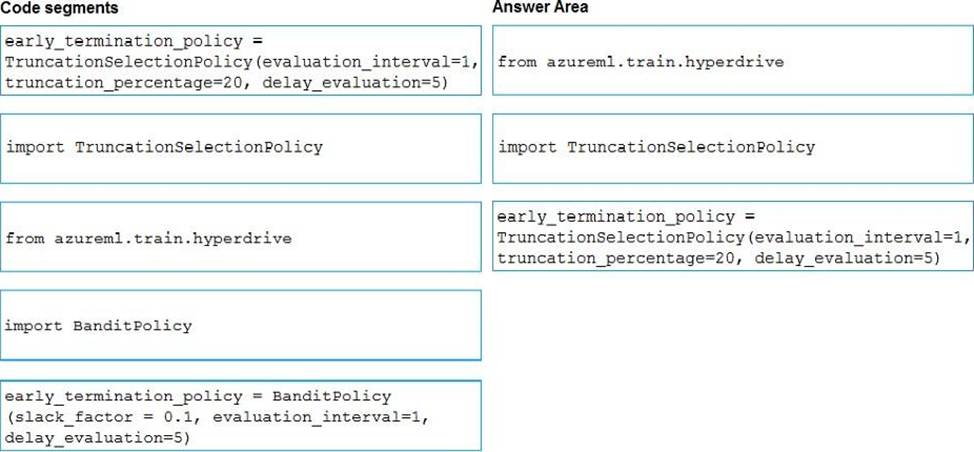
Explanation:
You need to implement an early stopping criterion on models that provides savings without terminating promising jobs.
Truncation selection cancels a given percentage of lowest performing runs at each evaluation interval. Runs are compared based on their performance on the primary metric and the lowest X% are terminated.
Example:
from azureml.train.hyperdrive import TruncationSelectionPolicy
early_termination_policy = TruncationSelectionPolicy(evaluation_interval=1, truncation_percentage=20, delay_evaluation=5)
Incorrect Answers:
Bandit is a termination policy based on slack factor/slack amount and evaluation interval. The policy early terminates any runs where the primary metric is not within the specified slack factor / slack amount with respect to the best performing training run.
Example:
from azureml.train.hyperdrive import BanditPolicy
early_termination_policy = BanditPolicy(slack_factor = 0.1, evaluation_interval=1, delay_evaluation=5
Reference: https://docs.microsoft.com/en-us/azure/machine-learning/service/how-to-tune-hyperparameters
HOTSPOT
You are developing a linear regression model in Azure Machine Learning Studio. You run an experiment to compare different algorithms.
The following image displays the results dataset output:
Use the drop-down menus to select the answer choice that answers each question based on the information presented in the image. NOTE: Each correct selection is worth one point.
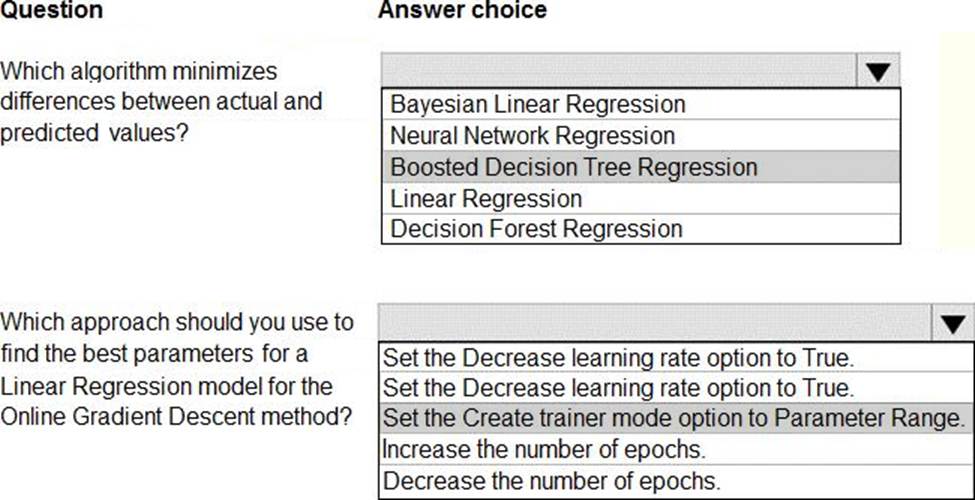
Explanation:
Box 1: Boosted Decision Tree Regression
Mean absolute error (MAE) measures how close the predictions are to the actual outcomes; thus, a lower score is better.
Box 2:
Online Gradient Descent: If you want the algorithm to find the best parameters for you, set Create trainer mode option to Parameter Range. You can then specify multiple values for the algorithm to try.
Reference:
https://docs.microsoft.com/en-us/azure/machine-learning/studio-module-reference/evaluate-model
https://docs.microsoft.com/en-us/azure/machine-learning/studio-module-reference/linear-regression
You create a binary classification model. The model is registered in an Azure Machine Learning workspace. You use the Azure Machine Learning Fairness SDK to assess the model fairness.
You develop a training script for the model on a local machine.
You need to load the model fairness metrics into Azure Machine Learning studio.
What should you do?
- A . Implement the download_dashboard_by_upload_id function
- B . Implement the creace_group_metric_sec function
- C . Implement the upload_dashboard_dictionary function
- D . Upload the training script
C
Explanation:
import azureml.contrib.fairness package to perform the upload:
from azureml.contrib.fairness import upload_dashboard_dictionary, download_dashboard_by_upload_id
Reference: https://docs.microsoft.com/en-us/azure/machine-learning/how-to-machine-learning-fairness-aml
Note: This question is part of a series of questions that present the same scenario. Each question in the series contains a unique solution that might meet the stated goals. Some question sets might have more than one correct solution, while others might not have a correct solution.
After you answer a question in this section, you will NOT be able to return to it. As a result, these questions will not appear in the review screen.
You are a data scientist using Azure Machine Learning Studio.
You need to normalize values to produce an output column into bins to predict a target column.
Solution: Apply a Quantiles normalization with a QuantileIndex normalization.
Does the solution meet the GOAL?
- A . Yes
- B . No
B
Explanation:
Use the Entropy MDL binning mode which has a target column.
Reference: https://docs.microsoft.com/en-us/azure/machine-learning/studio-module-reference/group-data-into-bins
You are moving a large dataset from Azure Machine Learning Studio to a Weka environment.
You need to format the data for the Weka environment.
Which module should you use?
- A . Convert to CSV
- B . Convert to Dataset
- C . Convert to ARFF
- D . Convert to SVMLight
C
Explanation:
Use the Convert to ARFF module in Azure Machine Learning Studio, to convert datasets and results in Azure Machine Learning to the attribute-relation file format used by the Weka toolset. This format is known as ARFF.
The ARFF data specification for Weka supports multiple machine learning tasks, including data preprocessing, classification, and feature selection. In this format, data is organized by entites and their attributes, and is contained in a single text file.
Reference: https://docs.microsoft.com/en-us/azure/machine-learning/studio-module-reference/convert-to-arff
HOTSPOT
You manage are Azure Machine Learning workspace by using the Python SDK v2.
You must create an automated machine learning job to generate a classification model by using data files stored in Parquet format. You must configure an auto scaling compute target and a data asset for the job.
You need to configure the resources for the job.
Which resource configuration should you use? to answer, select the appropriate options in the answer area. NOTE: Each correct selection is worth one point.
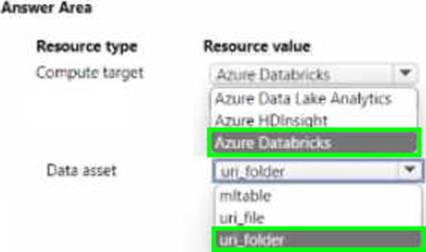
HOTSPOT
You manage are Azure Machine Learning workspace by using the Python SDK v2.
You must create an automated machine learning job to generate a classification model by using data files stored in Parquet format. You must configure an auto scaling compute target and a data asset for the job.
You need to configure the resources for the job.
Which resource configuration should you use? to answer, select the appropriate options in the answer area. NOTE: Each correct selection is worth one point.
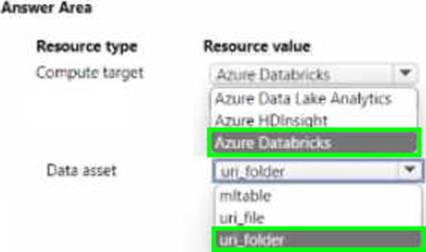
You are a lead data scientist for a project that tracks the health and migration of birds. You create a multi-class image classification deep learning model that uses a set of labeled bird photographs collected by experts.
You have 100,000 photographs of birds. All photographs use the JPG format and are stored in an Azure blob container in an Azure subscription.
You need to access the bird photograph files in the Azure blob container from the Azure Machine Learning service workspace that will be used for deep learning model training. You must minimize data movement.
What should you do?
- A . Create an Azure Data Lake store and move the bird photographs to the store.
- B . Create an Azure Cosmos DB database and attach the Azure Blob containing bird photographs storage to the database.
- C . Create and register a dataset by using TabularDataset class that references the Azure blob storage containing bird photographs.
- D . Register the Azure blob storage containing the bird photographs as a datastore in Azure Machine Learning service.
- E . Copy the bird photographs to the blob datastore that was created with your Azure Machine Learning
service workspace.
D
Explanation:
We recommend creating a datastore for an Azure Blob container. When you create a workspace, an Azure blob container and an Azure file share are automatically registered to the workspace.
Reference: https://docs.microsoft.com/en-us/azure/machine-learning/how-to-access-data