Practice Free DP-100 Exam Online Questions
HOTSPOT
You create an Azure Machine Learning dataset containing automobile price data The dataset includes 10,000 rows and 10 columns You use Azure Machine Learning Designer to transform the dataset by using an Execute Python Script component and custom code.
The code must combine three columns to create a new column.
You need to configure the code function.
Which configurations should you use? lo answer, select the appropriate options in the answer area. NOTE: Each correct selection is worth one point.
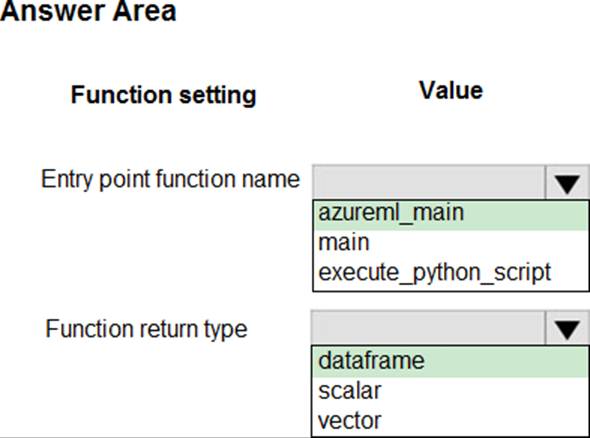
HOTSPOT
You are performing sentiment analysis using a CSV file that includes 12,000 customer reviews written in a short sentence format. You add the CSV file to Azure Machine Learning Studio and configure it as the starting point dataset of an experiment. You add the Extract N-Gram Features from Text module to the experiment to extract key phrases from the customer review column in the dataset.
You must create a new n-gram dictionary from the customer review text and set the maximum n-gram size to trigrams.
What should you select? To answer, select the appropriate options in the answer area. NOTE: Each correct selection is worth one point.
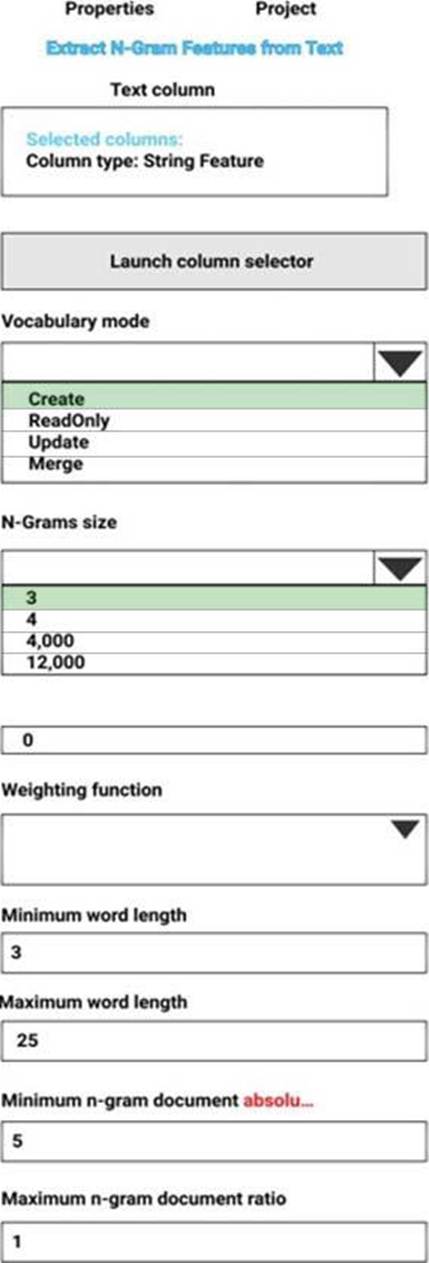
Explanation:
Vocabulary mode: Create
For Vocabulary mode, select Create to indicate that you are creating a new list of n-gram features.
N-Grams size: 3
For N-Grams size, type a number that indicates the maximum size of the n-grams to extract and store. For example, if you type 3, unigrams, bigrams, and trigrams will be created.
Weighting function: Leave blank
The option, Weighting function, is required only if you merge or update vocabularies. It specifies how terms in the two vocabularies and their scores should be weighted against each other.
Reference: https://docs.microsoft.com/en-us/azure/machine-learning/studio-module-reference/extract-n-gram-features-from-text
Note: This question is part of a series of questions that present the same scenario. Each question in the series contains a unique solution that might meet the stated goals. Some question sets might have more than one correct solution, while others might not have a correct solution.
After you answer a question in this section, you will NOT be able to return to it. As a result, these questions will not appear in the review screen.
You are creating a new experiment in Azure Machine Learning Studio.
One class has a much smaller number of observations than the other classes in the training set.
You need to select an appropriate data sampling strategy to compensate for the class imbalance.
Solution: You use the Stratified split for the sampling mode.
Does the solution meet the goal?
- A . Yes
- B . No
B
Explanation:
Instead use the Synthetic Minority Oversampling Technique (SMOTE) sampling mode.
Note: SMOTE is used to increase the number of underepresented cases in a dataset used for machine learning. SMOTE is a better way of increasing the number of rare cases than simply duplicating existing cases.
Reference: https://docs.microsoft.com/en-us/azure/machine-learning/studio-module-reference/smote
HOTSPOT
You plan to implement an Azure Machine Learning solution.
You have the following requirements:
• Run a Jupyter notebook to interactively tram a machine learning model.
• Deploy assets and workflows for machine learning proof of concept by using scripting rather than custom programming.
You need to select a development technique for each requirement
Which development technique should you use? To answer, select the appropriate options in the answer area. NOTE: Each correct selection is worth one point.
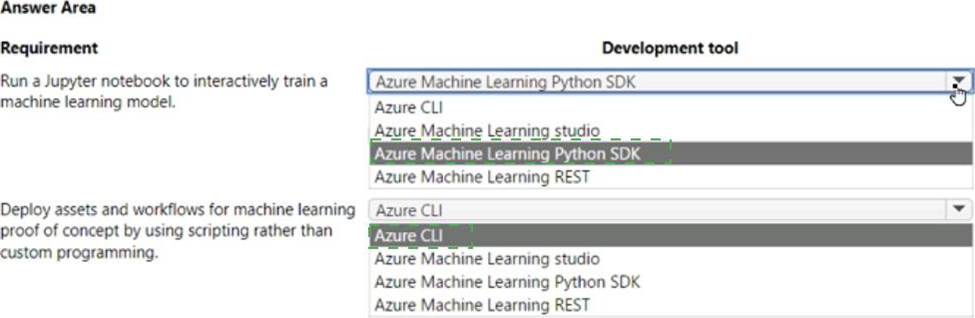
You create a classification model with a dataset that contains 100 samples with Class A and 10,000 samples with Class B
The variation of Class B is very high.
You need to resolve imbalances.
Which method should you use?
- A . Partition and Sample
- B . Cluster Centroids
- C . Tomek links
- D . Synthetic Minority Oversampling Technique (SMOTE)
HOTSPOT
You create an experiment in Azure Machine Learning Studio. You add a training dataset that contains 10,000 rows. The first 9,000 rows represent class 0 (90 percent).
The remaining 1,000 rows represent class 1 (10 percent).
The training set is imbalances between two classes. You must increase the number of training examples for class 1 to 4,000 by using 5 data rows. You add the Synthetic Minority Oversampling Technique (SMOTE) module to the experiment.
You need to configure the module.
Which values should you use? To answer, select the appropriate options in the dialog box in the answer area. NOTE: Each correct selection is worth one point.
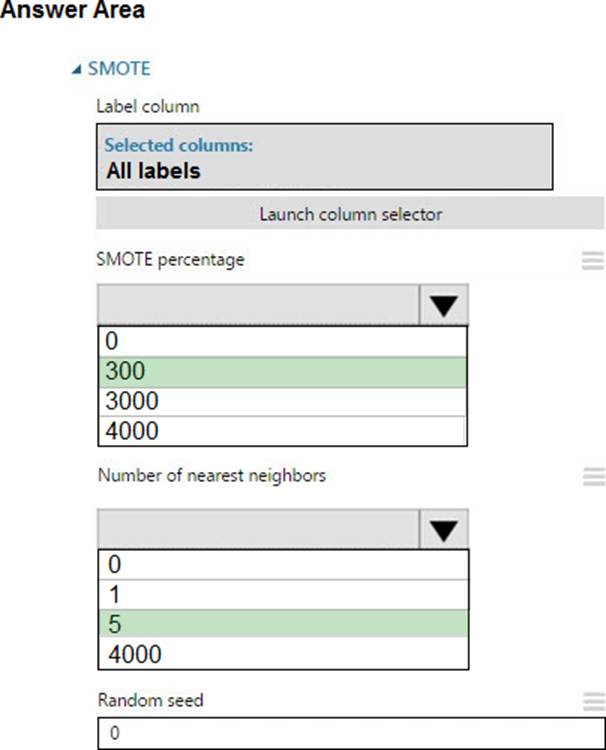
Explanation:
Box 1: 300
You type 300 (%), the module triples the percentage of minority cases (3000) compared to the original dataset (1000).
Box 2: 5
We should use 5 data rows.
Use the Number of nearest neighbors option to determine the size of the feature space that the SMOTE algorithm uses when in building new cases. A nearest neighbor is a row of data (a case) that is very similar to some target case. The distance between any two cases is measured by combining the weighted vectors of all features.
By increasing the number of nearest neighbors, you get features from more cases.
By keeping the number of nearest neighbors low, you use features that are more like those in the original sample.
Reference: https://docs.microsoft.com/en-us/azure/machine-learning/studio-module-reference/smote
Note: This question is part of a series of questions that present the same scenario. Each question in the series contains a unique solution that might meet the stated goals. Some question sets might have more than one correct solution, while others might not have a correct solution.
After you answer a question in this section, you will NOT be able to return to it. As a result, these questions will not appear in the review screen.
You are a data scientist using Azure Machine Learning Studio.
You need to normalize values to produce an output column into bins to predict a target column.
Solution: Apply a Quantiles binning mode with a PQuantile normalization.
Does the solution meet the goal?
- A . Yes
- B . No
B
Explanation:
Use the Entropy MDL binning mode which has a target column.
Reference: https://docs.microsoft.com/en-us/azure/machine-learning/studio-module-reference/group-data-into-bins
Note: This question is part of a series of questions that present the same scenario. Each question in the series contains a unique solution that might meet the stated goals. Some question sets might have more than one correct solution, while others might not have a correct solution.
After you answer a question in this section, you will NOT be able to return to it. As a result, these questions will not appear in the review screen.
You are a data scientist using Azure Machine Learning Studio.
You need to normalize values to produce an output column into bins to predict a target column.
Solution: Apply a Quantiles binning mode with a PQuantile normalization.
Does the solution meet the goal?
- A . Yes
- B . No
B
Explanation:
Use the Entropy MDL binning mode which has a target column.
Reference: https://docs.microsoft.com/en-us/azure/machine-learning/studio-module-reference/group-data-into-bins
Note: This question is part of a series of questions that present the same scenario. Each question in the series contains a unique solution that might meet the stated goals. Some question sets might have more than one correct solution, while others might not have a correct solution.
After you answer a question in this section, you will NOT be able to return to it. As a result, these questions will not appear in the review screen.
You create a model to forecast weather conditions based on historical data.
You need to create a pipeline that runs a processing script to load data from a datastore and pass the processed data to a machine learning model training script.
Solution: Run the following code:
Does the solution meet the goal?
- A . Yes
- B . No
B
Explanation:
train_step is missing.
Reference: https://docs.microsoft.com/en-us/python/api/azureml-pipeline-core/azureml.pipeline.core.pipelinedata?view=azure-ml-py
You are creating a binary classification by using a two-class logistic regression model.
You need to evaluate the model results for imbalance.
Which evaluation metric should you use?
- A . Relative Absolute Error
- B . AUC Curve
- C . Mean Absolute Error
- D . Relative Squared Error
B
Explanation:
One can inspect the true positive rate vs. the false positive rate in the Receiver Operating Characteristic (ROC) curve and the corresponding Area Under the Curve (AUC) value. The closer this curve is to the upper left corner, the better the classifier’s performance is (that is maximizing the true positive rate while minimizing the false positive rate). Curves that are close to the diagonal of the plot, result from classifiers that tend to make predictions that are close to random guessing.
Reference: https://docs.microsoft.com/en-us/azure/machine-learning/studio/evaluate-model-performance#evaluating-a-binary-classification-model